Labeling Training Data for Improved Business Efficiency
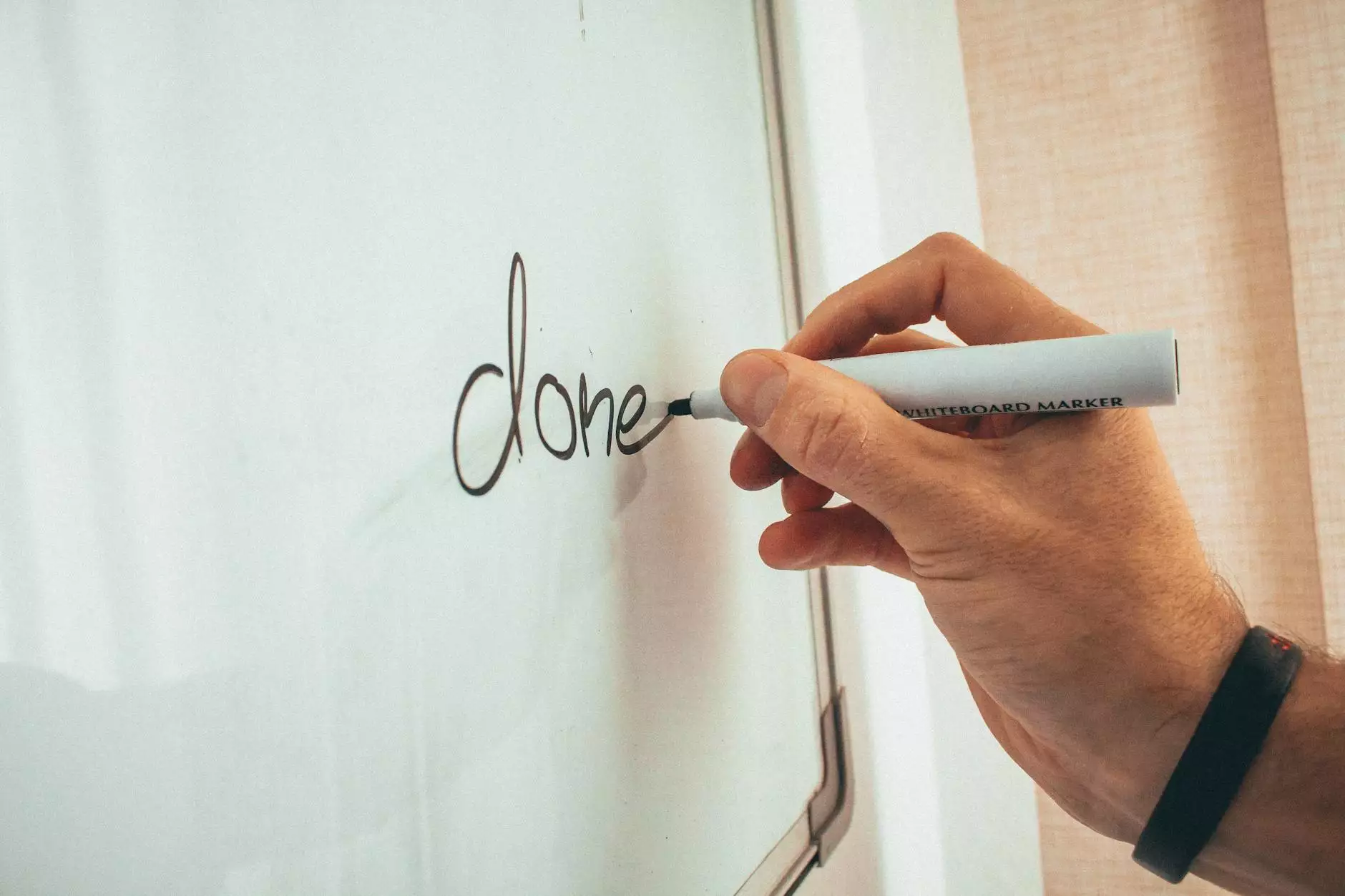
Introduction
In today's digital age, businesses in the domain of IT services & computer repair face numerous challenges, especially when it comes to accurately categorizing and analyzing vast amounts of data. This is where advanced techniques like labeling training data come into play.
The Importance of Labeling Training Data
Labeling training data is the process of assigning tags or categories to the datasets used to train machine learning models. It plays a pivotal role in driving accurate predictions and enhancing the efficacy of computer repair services.
Properly labeled data ensures that businesses can leverage automation and artificial intelligence to provide efficient solutions, leading to improved overall customer satisfaction and operational performance.
The Role of Labeling Training Data in IT Services
IT services companies like keylabs.ai have recognized the immense potential of labeling training data to optimize their operations. By categorizing and organizing data, businesses can streamline their processes, identify patterns, and make informed business decisions.
Effective data labeling enables computer repair businesses to automate various tasks, such as identifying software bugs, diagnosing hardware issues, and recommending appropriate solutions. The accuracy and efficiency of these automated processes directly impact customer convenience and satisfaction.
Enhancing Data Quality through Labeling
Unstructured, unlabeled data can hinder a business's ability to operate at its fullest potential. By leveraging labeling training data methodologies, companies can transform raw data into structured and meaningful information.
Labeling data unlocks the capability to train machine learning algorithms that can identify patterns, classify issues, and predict outcomes. This, in turn, allows IT service providers to offer more reliable services, reducing downtime, and providing faster and more accurate solutions to their customers.
Best Practices for Labeling Training Data
Successful labeling of training data requires careful consideration and adherence to specific best practices:
1. Define Clear Labeling Guidelines
Establishing precise and easily understandable guidelines for labeling data ensures consistency throughout the process. This consistency is critical, as it directly impacts the accuracy and reliability of the trained models.
2. Utilize Expertise and Domain Knowledge
Collaboration between domain experts and data annotators can significantly improve the quality of labeling. Domain experts possess valuable insights and contextual knowledge necessary for labeling data accurately.
3. Employ Iterative Labeling Approaches
Large datasets may require iterative labeling approaches, involving multiple rounds of annotation and verification. This iterative process allows for continuous refinement, leading to more accurate and reliable data.
4. Leverage Automation and AI-Assisted Labeling
Artificial intelligence can greatly expedite the labeling process by automatically suggesting labels based on pre-trained models. This streamlines the process, reduces human effort, and enhances efficiency.
Conclusion
Accurate and well-labeled training data is the foundation for successful IT services & computer repair businesses. By leveraging advanced labeling techniques, such as the labeling training data methodology, companies can unlock the true potential of automation, artificial intelligence, and machine learning.
Keylabs.ai is at the forefront of utilizing labeling training data to enhance business efficiency, optimize repairs, and deliver exceptional customer experiences. Incorporating meticulous data labeling practices aids in providing reliable solutions, reducing downtime, and ultimately outperforming competitors in the industry.